Two-Minute Tech
The healthcare industry is using AI to transform drug discovery
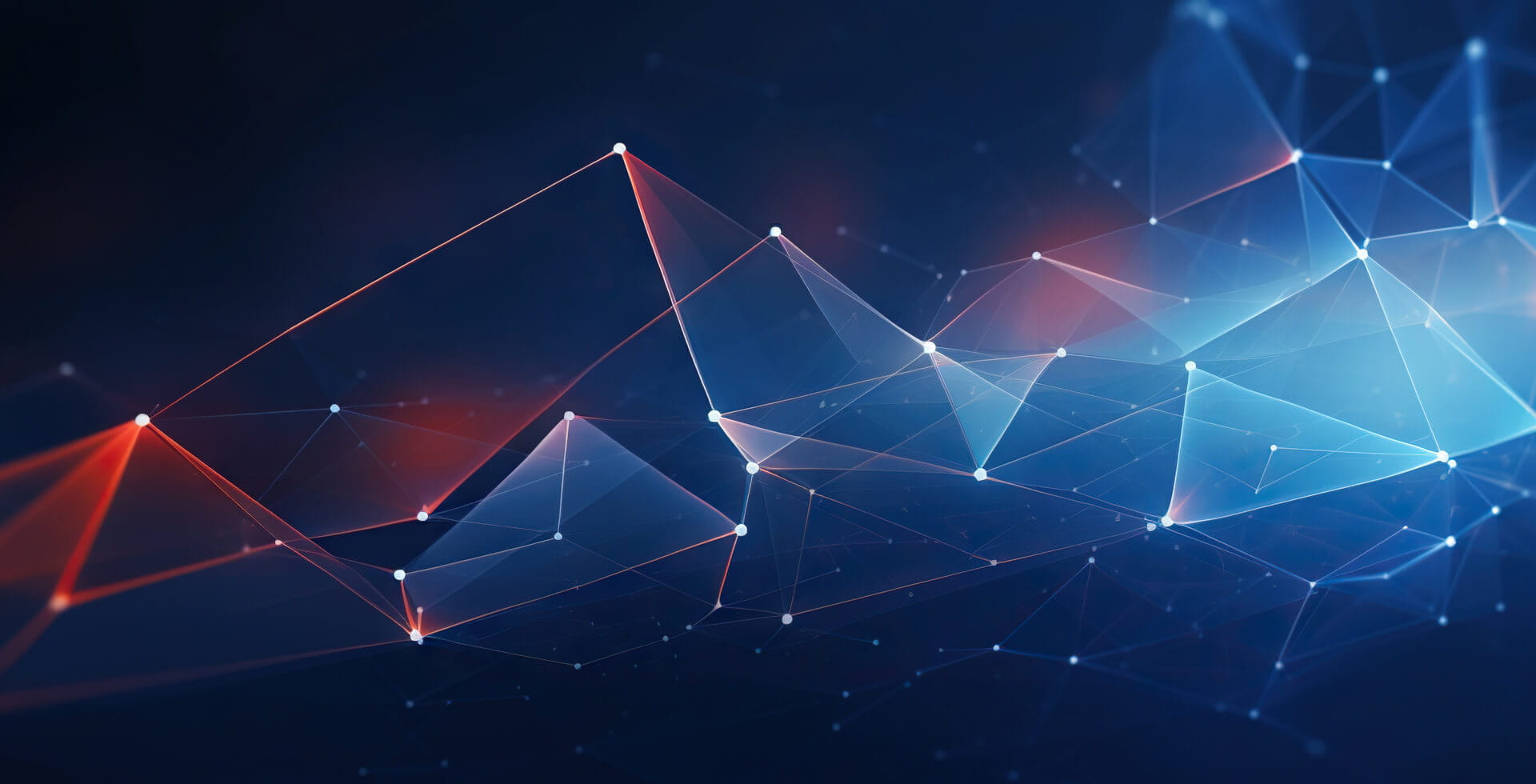
Amid increasing calls for robust regulations, AI is more about streamlining work processes than replacing human involvement, making it an indispensable tool in the pharmaceutical industry.
- Artificial-intelligence (AI)-based drug design can significantly accelerate drug discovery, enhance product quality and cut costs of clinical trials by targeting key failure points in critical stages of drug discovery processes
- One large pharmaceutical company uses AI to minimise the number of experimental trials, with the aim of reducing synthesis lead times from 9-12 months to less than 3 months
- Amid increasing calls for robust regulations, it’s important to remember that AI is more about streamlining work processes than replacing human involvement, making it an indispensable tool in the pharmaceutical industry
How the healthcare industry is transforming drug discovery with AI
The healthcare ecosystem overall has made slow progress adopting innovative, productivity-enhancing technologies – largely due to the challenges associated with operating in a highly regulated environment. But innovative solutions are desperately needed, particularly in the United States, where healthcare costs in 2022 (the latest figures available) reached a staggering USD 4.5 trillion, or 17.3% of GDP1.
On a global scale, the costs for launching a new medicine on the market can amount to far above 1 trillion USD and take up to 14 years2. In parallel, the failure rate in clinical trials is extremely high, currently reaching 90%3.
One factor driving these high costs is the increasing prevalence of chronic conditions and the elevated expenses associated with their treatment. But artificial intelligence in general, and more specifically, AI-backed drug design is already fundamentally reshaping the landscape of drug discovery, providing powerful (software) tools that effectively address the common challenges encountered by pharmaceutical companies.
Pharmaceutical manufacturing uses AI to facilitate the production of customised medications, tailored to the specific needs of individual patients, encompassing personalised dosages and precise release specifications. The application of cutting-edge AI-based technologies not only accelerates product development but also enhances product quality, production safety, resource utilisation and cost-effectiveness, underscoring the mounting importance of automation.
Among the many use cases:
- Identifying promising compounds while providing insights into synthesis routes, chemical structure predictions and drug-target interactions
- Optimising drug dosages, expediting decision-making and facilitating the efficient production of high-quality products with consistent performance across batches
- Verifying product safety and efficacy during clinical trials
- Ensuring effective market positioning and pricing by conducting comprehensive market analyses and predictions
AI-developed drugs have yet to appear in the market, and certain challenges remain in adopting this technology. There are also concerns about potential job displacement and the necessity for robust AI regulations. But it’s important to emphasise that overall, AI is being used to streamline work processes, rather than entirely replace human involvement. As such, AI is on the verge of becoming an indispensable tool in the pharmaceutical industry.
Examples of drug companies using AI in innovative ways
A leading diabetes management company (DMC) has been at the forefront of expanding the commercial reach of continuous glucose monitoring systems for people with diabetes — systems that measure glucose levels beneath the skin and relay this information to smart devices or the cloud. The company’s latest product launch incorporates AI to ensure the precision and reliability of sensor readings and the underlying algorithm. Notably, a recent study demonstrated that using these systems resulted in a substantial reduction (of USD 424 per person per month, on average) in the cost of managing type 2 diabetes4. This reduction is primarily attributed to a decrease in inpatient medical costs related to diabetes, as patients experienced fewer expensive hospital admissions and shorter stays.
A large-cap pharmaceutical company (LPC) is actively striving to be at the forefront of deploying AI across various aspects of their operations. Among the company’s more notable initiatives:
- The company uses a proprietary AI-enabled tool in drug development to minimise the number of experimental trials. So far, LPC has substantially reduced synthesis lead times from 9-12 months to 5-6 months — with an ultimate goal of less than 3 months5.
- It harnessed AI-powered data visualisation to enhance the performance of synthetic and biological medicine processes, making real-time optimisation adjustments that lead to improved yields and cost savings.
- The company also employs AI-powered digital twins that can simultaneously leverage multiple data sources to optimise production schedules, resulting in a remarkable 90% improvement6.
The bottom line
Given the pharmaceutical industry’s declining research and development productivity over recent decades, leveraging AI in drug development holds great promise. It can expedite the identification of more effective compounds, reduce development timelines for successful products, lower costs associated with less promising compounds and ultimately enhance returns on investment.
Historically, the healthcare sector has not been known for its rapid adoption of new technologies across organisations. But it is becoming increasingly evident that AI technologies are now being embraced by healthcare leaders on a broad scale to address their respective markets and enhance overall business performance. This reflects a transformative shift in how healthcare is leveraging technology to meet the evolving needs of the industry.
1 Centers for Medicare and Medicaid Services, National Health Expenditures 2022 Highlights. As of 31 December 2023.
2 National library of medicine: AI in drug discovery and its clinical relevance. As of June 2023.
3 MIT News: Speeding up drug discovery with diffusion generative models. As of May 2023.
4 “Liebert Publishers: Real-Time Continuous Glucose Monitor Glucose Monitor Use Associated with Decreased Diabetes Medical Costs for Patients with Type 2 Diabetes”, as of June 2021.
5 Company earnings call as of July 2023.
6 Company earnings call as of July 2023.